Deploying AI Agents for Intelligent Automation: A Comprehensive Guide
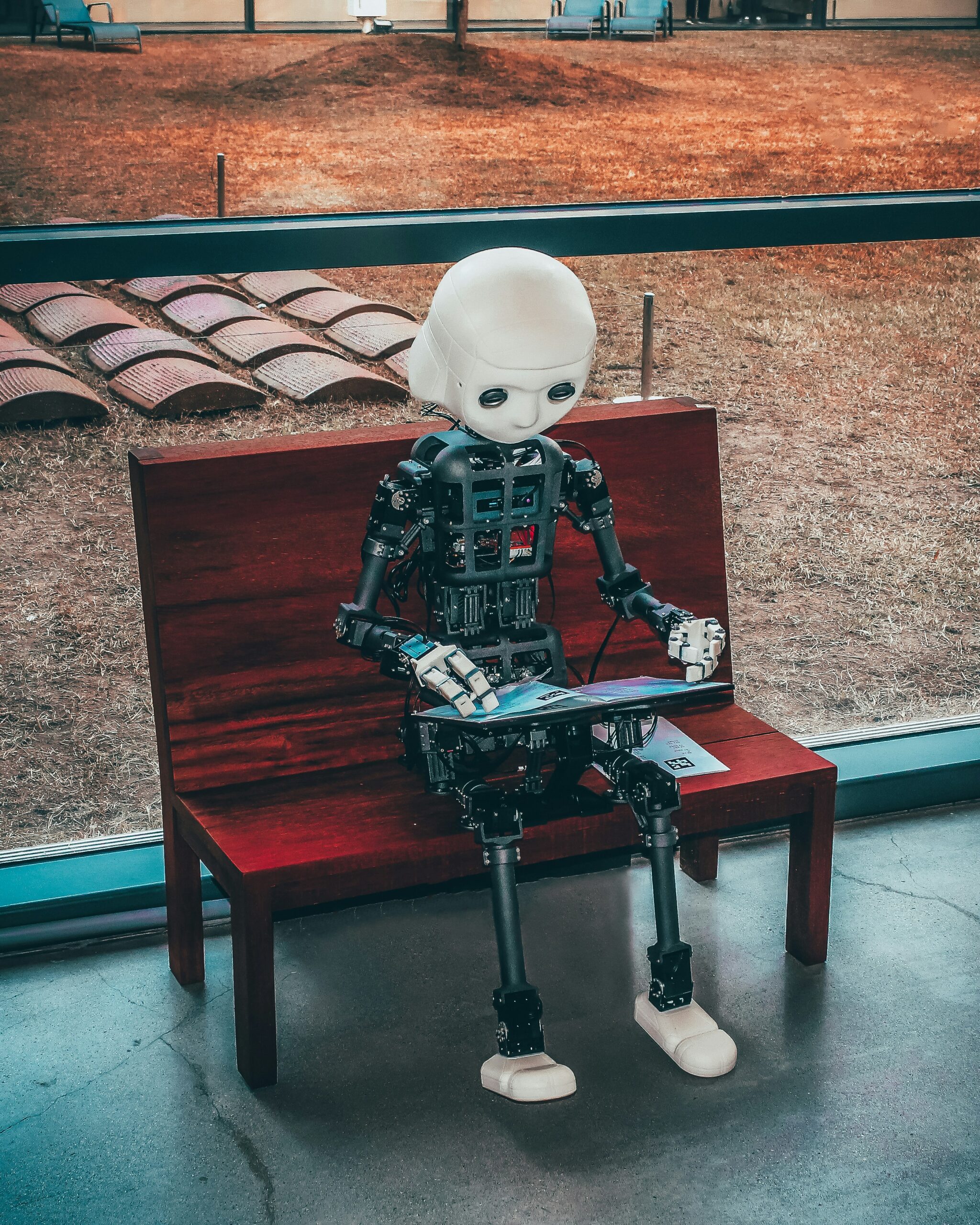
Introduction to AI Agents and Intelligent Automation
Artificial Intelligence (AI) agents play a pivotal role in the evolution of intelligent automation, making them increasingly essential in various sectors. An AI agent is a software entity designed to perceive its environment, process data, and take action to achieve specific goals with minimal human intervention. These agents utilize advanced machine learning and data analysis techniques to optimize processes, streamline operations, and respond to changes swiftly and effectively. This capability is particularly crucial as industries face heightened pressure to enhance productivity and efficiency, leading to the burgeoning demand for automation solutions.
Various industries, including finance, healthcare, manufacturing, and logistics, are recognizing the necessity for automation to stay competitive in today’s fast-paced digital landscape. Traditional automation methods often rely on predefined rules and manual programming, which can be limiting. In contrast, AI-driven approaches empower agents to learn from data and adapt to new situations, enabling them to handle complex tasks and make informed decisions. This transformation facilitated by AI agents can result in increased operational efficiency, reduced costs, and improved service quality.
While traditional automation can optimize repetitive tasks, it lacks the capability to adjust autonomously in dynamic environments. AI agents, however, combine automation with cognitive abilities, allowing them to analyze vast amounts of data, predict outcomes, and adjust processes in real-time. This distinction not only enhances productivity but also fosters innovation within organizations, as teams can focus on higher-value initiatives rather than getting bogged down by routine tasks. Understanding the principles behind AI agents is fundamental to appreciating their potential role in intelligent automation. This guide will delve deeper into the deployment process of these agents in later sections, providing insights into best practices and strategies for successful integration into existing systems.
Identifying Opportunities for AI Automation
Identifying opportunities for AI automation within business processes is a critical first step towards leveraging intelligent automation effectively. Organizations must assess their workflows to discern which tasks can be enhanced or entirely automated using AI agents. One effective approach is to conduct a comprehensive analysis of all current operations. It involves mapping out processes to visualize where inefficiencies or bottlenecks occur, revealing areas ripe for improvement.
Several techniques can be employed in this assessment. Value stream mapping is one such method that enables teams to analyze each step in a process, pinpointing those that do not contribute value. Through careful examination, organizations can identify repetitive, labor-intensive tasks that hinder productivity. These tasks are often prime candidates for automation, as AI agents can manage them with enhanced efficiency and accuracy.
When assessing workflows, it is essential to consider data availability. The effectiveness of AI depends significantly on the quality and quantity of data it can process. Therefore, businesses should evaluate whether they possess the necessary data to train AI agents adequately. Additionally, process complexity should be factored in; simpler and more predictable tasks are generally easier to automate. Organizations should also weigh the expected return on investment (ROI) from automation initiatives. Conducting a cost-benefit analysis will help in understanding the financial implications and potential gains from implementing AI-driven solutions.
Moreover, it is beneficial to involve various stakeholders in the evaluation process. Input from employees who perform the tasks on the ground can provide valuable insights into existing challenges and obstacles. By carefully considering these factors and leveraging systematic approaches, organizations can identify the most suitable processes for AI automation, paving the way for a successful deployment of AI agents.
Steps to Deploy AI Agents Effectively
Deploying AI agents for intelligent automation requires a structured approach that ensures the alignment of technology with business objectives. The first step involves the design and development of AI solutions tailored to the specific needs of the organization. This entails a thorough needs analysis to identify processes that could benefit from automation. Engaging stakeholders from both business and IT teams at this stage is crucial, as it fosters a collaborative environment that enhances the workflow and results in more relevant solutions.
Once the requirements are established, the next step is to select appropriate tools and technologies. There are numerous frameworks and platforms available, each with distinct features and capabilities suited for different types of AI applications. It is important to consider scalability, compatibility with existing systems, and vendor reliability during this selection process. The decision should not solely focus on current needs; rather, it should consider future growth and potential advancements in AI technologies.
Integration of AI agents with existing systems is another vital step. This process may involve configuring APIs and ensuring that data flows seamlessly between the AI agents and the current software infrastructure. Collaboration between IT and business teams plays a pivotal role here, as technical personnel must understand the operational requirements of business teams to facilitate smooth integration.
Subsequently, testing and monitoring are essential to validate the performance of AI agents post-deployment. A robust testing strategy should be devised to assess the AI model’s accuracy, efficiency, and capability to handle real-time data. Continuous monitoring allows teams to gather insights about AI performance, enabling necessary adjustments and improvements to enhance efficiency. This iterative approach guarantees that AI agents evolve along with the business landscape, maintaining optimal performance and maximizing the benefits of intelligent automation.
Case Studies and Best Practices in AI Agent Deployment
Deploying AI agents in various industries has provided valuable insights and lessons learned on the utilization of intelligent automation. One notable case study is that of a global retail chain, which integrated AI agents to streamline their inventory management system. By implementing machine learning algorithms, the retailer was able to optimize stock levels, predict demand fluctuations, and automate ordering processes. Consequently, this led to a significant reduction in holding costs and enhanced overall operational efficiency. The deployment of AI agents resulted in a documented 20% improvement in stock accuracy and a 15% increase in customer satisfaction due to timely product availability.
Another impactful example comes from the financial services sector, where a major bank leveraged AI agents to enhance customer service through intelligent chatbots. By deploying these conversational agents, the bank successfully managed a high volume of inquiries. With capabilities such as natural language processing, the AI agents offered instant responses for common queries, freeing human agents to focus on more complex issues. This implementation not only reduced operating costs by approximately 30% but also enhanced customer engagement, leading to higher retention rates. Best practices from this project emphasize the importance of continuous learning and adapting the AI models based on customer interactions to ensure relevance and accuracy.
Furthermore, the manufacturing sector illustrates the benefits of AI agents in predictive maintenance. A leading manufacturer adopted AI-driven analytics to monitor machinery performance, predicting failures before they occurred. This proactive approach minimized downtime and repair costs, illustrating the viability of intelligent automation in safeguarding operational integrity. Critical lessons from this deployment include the significance of a robust data architecture and the need for integration with existing systems to optimize AI agent performance.
In looking to the future, emerging trends in AI agent technology suggest ongoing advancements in natural language understanding and machine learning capabilities, potentially broadening the applications of intelligent automation across diverse sectors. By staying informed about these evolving trends, organizations can better position themselves to harness the power of AI agents.